This blog is about ‘Big Data’. I think it’s worth understanding what’s happening within this space, and my aim is to give a (reasonably) short introduction to the concept and possible relevance for archives.
I attended the the Eduserv Symposium 2012 on Big Data , and this post is partly inspired by what I heard there, in particular the opening talk by Rob Anderson, Chief Technology Officer EMEA. Thanks also to Adrian Stevenson and Lukas Koster for their input during our discussions of this topic (over a beer!).
What is Big Data? In the book Planning for Big Data (Edd Dumbill, O’Reilly) it is described as:
“data that exceeds the processing capacity of conventional database systems. The data is too big, moves too fast, or doesn’t fit the strictures of your database architectures. To gain value from this data you must choose an alternative way to process it.”
Big data is often associated with the massive and growing scale of data, and at the Eduserv Symposium many speakers emphasised just how big this increase in data is (which got somewhat repetitive). Many of them spoke about projects that involve huge, huge amounts of data, in particular medical and scientific data. For me tera- and peta- and whatever else -bytes don’t actually mean much. Suffice to say that this scale of data is way way beyond the sort of scale of data that I normally think about in terms of archives and archive descriptions.
We currently have more data than we can analyse, and 90% of the digital universe is unstructured, a fact that drives the move towards the big data approach. You may think big data is new; you may not have come across it before, but it has certainly arrived. Social media, electronic payments, retail analytics, video analysis of customers, medical imaging, utilities data, etc, etc., all are in the Big Data space, and the big players are there too – Google, Amazon, Walmart, Tesco, Facebook, etc., etc., – they all stand to gain a great deal from increasingly effective data analysis.
Very large scale unstructured data needs a different approach to structured data. With structured data there is a reasonable degree of routine. Data searches of a relational database, for example, are based around the principle of searching specific fields, the scope is already set out. But unstructured data is different, and requires a certain amount of experimentation with analysis.
The speakers throughout the Eduserv symposium emphasised many of the benefits that can come with the analysis of unstructured data. For example, Rob Anderson argued that we can raise the quality of patient care through analysis of various data sources – we can take into account treatments, social and economic factors, international perspective
, individual patient history. Another example he gave was the financial collapse: could we have averted this at least to some extent through being able to identify ‘at risk’ customers more effectively? It certainly seems convincing that analysing and understanding data more thoroughly could help us to improve public services and achieve big savings. In other words, data science principles could really bring public benefits and value for money.
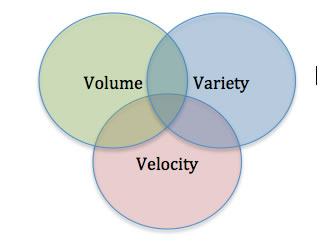
But the definition of big data as unstructured data is only part of the story. It is often characterised by three things, volume (scale), velocity (speed) and variety (heterogenous data).
It is reasonably easy to grasp the idea of volume – processing huge quantities of data. For this scale of data the traditional approaches, based on structured data, are often inadequate.
Velocity may relate to how quickly the data comes into the data centre and the speed of response. Real-time analysis is becoming increasingly effective, used by players like Google. It enables them to instantly identify and track trends – they are able to extract value from the data they are gathering. Similarly Facebook and Twitter are storing every message and monitoring every market trend. Amazon react instantly to purchase information – this can immediately affect price and they can adjust the supply chain. Tesco, for example, know when more of something is selling intensively and can divert supplies to meet demand. Big data approaches are undoubtedly providing great efficiencies for companies, although it raises the whole question of what these companies know about us and whether we are aware of how much data we are giving away.
The variety of data refers to diversity, maybe data from a variety of sources. It may be un-curated, have no schema, be inconsistent and changing. With these problems it can be hard to extract value. The question is, what is the potential value that can be extracted and how can that value be used to good effect? It may be that big data leads to the opti
mised organisation, but it takes time and skill to build what is required and it is important to have a clear idea of what you are wanting to achieve – what your value proposition is. Right now decisions are often made based on pretty poor information, and so they are often pretty poor decisions. Good data is often hard to get, so decisions may be based on little or no data at all. Many companies fail to detect shifts in consumer demand, but at the same time the Internet has made customers more segmented, so the picture is more complex. Companies need to adjust to this and respond to differing requirements. They need to take a more scientific approach because sophisticated analytics makes for better decisions and in the end better products.
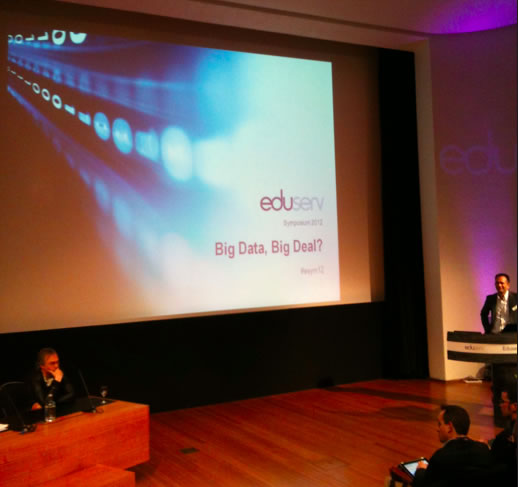
At the Eduserv Symposium there were a number of speakers who provided some inspirational examples of what is possible with big data solutions. Dr Guy Coates from the Wellcome Trust Sanger Institute talked about the human genome. The ability to compare, correlate with other records and work towards finding genetic causes for diseases opens up exciting new opportunities. It is possible to work towards more personalised medicine, avoiding the time spent trying to work out which drugs work for individuals. Dr Coates talked about the rise of more agile systems, able to cope with this way of working, more modular design and an evolving incremental approach rather than the typical 3-year cycle of complete replacement of hardware.
Professor Anthony Brookes from the University of Leicester introduced the concept of ‘knowledge engineering’, thinking about this in the context of health. He stated that in many cases it may be that the rate of data generation is increasing, but it is often the same sort of data, so it may be that scale is not such an issue in all cases. This is an important point. It is easy to equate big data with scale, but it is not all about scale. The rise of Big Data is just as concerned with things like new tools that are making analysis of data more effective.
Prof Brookes described knowledge engineering as a discipline that involves integrating knowledge into computer systems in order to solve complex problems (see the i4health website for more information). He effectively conveyed how we have so much medical knowledge, but the knowledge is simply not used properly. Research and healthcare are separate – the data does not flow properly between them. We need to bring together bio-informatics and academics with medical informatics and companies, but at the moment there is a very definite gap, and this is a really really big problem. We need to build a bridge between the two, and for this you need an engineer – a knowledge engineer – someone with expertise to work through the issues involved in bridging the gap and getting the data flow right. The knowledge engineer needs to understand the knowledge potential, understand standards, understand who owns the data, understand the ethics, think about what is required to share data, such as having researcher IDs, open data discovery, remote pooled analysis of data, categories of risk for data. This type of role is essential in order to effect an integration of data with knowledge.
As well as hearing about the knowledge engineer we heard about the rise of the ‘data scientist‘, or, rather more facetiously, the “business analyst who lives in Califorina” (Adam Cooper’s blog). This concept of a data scientist was revisited throughout the Eduserv symposium. At one point it was referred to as “someone who likes telling stories around data”, an idea that immediately piqued my interest. It did seem to be a broad concept, encompassing both data analysis and data curation, although in reality these roles are really quite distinct, and there was acknowledgement that they need to be more clearly defined.
Big data has helped to create an atmosphere where expectations people have of the public sector are no longer met; expectations created by what is often provided in the private sector, for example, a more rapid response to enquiry. We expect the commercial sector to know about us and understand what we want and maybe we think public services should do the same? But organisational change is a big challenge in the public sector. Data analysis can actually be seen as opposed to the ‘human agenda’ as it moves away from the principle of human relationships. But data can drive public service innovation, and help to allocate resources efficiently, in a way that responds to need.
Big Data raises the question of the benefits of open, transparent and shared information. This message seems to come to the fore again and again, not just with Big Data, but with the whole open data agenda and Linked Data area. For example, advance warning for earthquakes requires real-time analytics – it is hard to extract this information from the diverse systems that are out there. But a Twitter-based Earthquake Detector provides substantial benefits. Simply by following #quake tweets it is possible to get a surprisingly accurate picture very quickly; apparently more #quake tweets has been shown to be an accurate indication of a bigger quake. Twitter users reacted extremely quickly to the quake and tsunami in Japan. In the US, the Government launched a cash for old cars initiative (Cash for Clunkers), to encourage people to move to greener vehicles. The Government were not sure whether the initiative was proving to be successful, but Google knew that it was, because they had the information about what people were searching for and they could see people searching for this initiative to find information about what the Government were offering. Google can instantly find out where in the world people are searching for information – for example, information about flu trends, because they can analyse which search terms are relevant, something called ‘nowcasting‘.
In the commercial sector big data is having a big impact, but it is less certain what its impact is within higher education and sectors such as cultural heritage. The question many people seem to be asking is ‘how will this be relevant to us?’
Big data may have implications for archivists in terms of what to keep and what to delete. We often log everything because we don’t know what questions we will want the answer to. But if we decide we can’t keep everything then what do we delete? We know that people only tend to criticise if you get it wrong. In the US the new National Science Foundation data retention requirements now mean you have to keep all data for 3 years after the research award conclusion, and you must produce a data management plan. But with more and more sophisticated means of processing data, should we be looking to keep data that we might otherwise dispose of? We might have considered it expensive to manage and hard to extract value from, but this is now changing. Should we always keep everything when we can? Many companies are simply storing more and more data ‘just in case’; partly because they don’t want to risk being accused of throwing it away if it turns out to be important. Our ideas of what we can extract from data are changing, and this may have implications for its value.
Does the archive community need to engage with big data? At the Eduserv Symposium one of the speakers referred to NARA making available documents for analysis. The analysis of data is something that should interest us:
“A common use of big data processing is to take unstructured data and extract ordered meaning, for consumption either by humans or as a structured input to an application.” (Planning for Big Data, Edd Dumbill).
For example, you might be looking to determine exactly what a name refers to: is this city London, England or London, Texas? Tasks such as crowd-sourcing, cleaning data, answering imprecise questions, these are relevant in the big data space and therefore potentially relevant within archives.
As already stated, it is about more than scale, and it can be very relevant to the end-user experience: “Big data is often about fast results, rather than simply crunching a large amount of information.” (Dumbill) For example, the ability to suggest on-the-fly which books someone might enjoy requires a system to provide an answer in the time it takes a page to load. It is possible to think about ways that this type of data processing could enhance the user experience within the archives space; helping the user to find what might be of relevance to their research. Again, expectations may start to demand that we provide this type of experience, as many other information sites already provide it.
The Eduserv Symposium concluded that there is a skills gap in both the public and commercial sector when it comes to big data. We need a new generation of “big data scientists” to meet a need. We also need to combine algorithms, machines and people holistically to meet the big data problem. There may be an issue around mindset, particularly in terms of worries about the use of data – something that arises in the whole open data agenda. In addition, one of the big problems we have at the moment is that we are often building on infrastructures that are not really suited to this type of unstructured data. It takes time, and knowledge of what is required, to move towards a new infrastructure, and the advance of big data may be held back by the organisational change required and skills needed to do this work.